The accuracy of climate model predictions is influenced by various factors including data quality and availability, model complexity and resolution, initial conditions and parameterizations, natural variability and external forcing, and the use of model intercomparison and ensemble methods. High-quality, up-to-date data and comprehensive models that account for multiple physical processes and high-resolution details are crucial. Initial conditions, sub-grid scale process parameterizations, internal climate variability, and external forcing factors add layers of complexity and uncertainty. To mitigate these uncertainties, scientists employ intercomparison projects and ensemble forecasting techniques to assess model reliability and potential future climate scenarios.
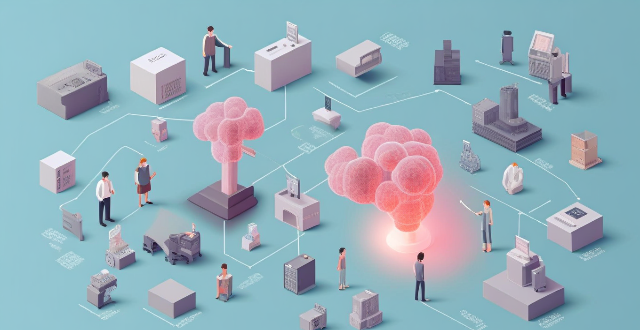
Factors Affecting the Accuracy of Climate Model Predictions
Climate models are complex tools used to predict future climate conditions based on current and historical data. The accuracy of these predictions can be influenced by a variety of factors, which we will explore in detail below.
1. Data Quality and Availability
The quality and availability of data play a crucial role in the accuracy of climate model predictions. If the data used to build the model is incomplete or inaccurate, it can lead to significant errors in the predictions. This includes:
- Historical Climate Data: Gaps or inconsistencies in historical climate records can make it difficult to establish accurate baselines for future projections.
- Current Environmental Conditions: Up-to-date measurements of atmospheric composition, ocean temperatures, and other variables are essential for creating realistic models.
- Spatial Resolution: Higher resolution data allows for more detailed analysis, improving the model's ability to capture localized effects and feedback mechanisms.
2. Model Complexity and Resolution
The complexity and resolution of the climate model itself also impact its predictive accuracy:
- Physical Processes Included: More comprehensive models that incorporate a wide range of physical processes (e.g., atmospheric dynamics, ocean circulation, and biogeochemical cycles) generally provide more accurate simulations.
- Numerical Resolution: Higher numerical resolution enables the model to better represent small-scale features and processes, which can be important for regional climate predictions.
3. Initial Conditions and Parameterizations
The initial conditions set for the model and the way certain processes are parameterized within the model can significantly affect the outcomes:
- Starting Point: Different starting points may lead to divergent climate trajectories due to the chaotic nature of the climate system.
- Sub-Grid Scale Processes: Since not all processes can be resolved at the model's grid scale, they must be parameterized, which introduces additional uncertainties.
4. Natural Variability and External Forcing
Natural variability within the climate system as well as external forcing factors contribute to uncertainty in model predictions:
- Internal Climate Variability: Phenomena like El Niño Southern Oscillation (ENSO) can cause significant year-to-year fluctuations in regional climates.
- External Forcing Factors: Changes in solar radiation, volcanic eruptions, and human activities (such as greenhouse gas emissions) can perturb the climate system in ways that are challenging to predict accurately.
5. Model Intercomparison and Ensemble Methods
To address some of these uncertainties, scientists often use multiple models and ensemble techniques:
- Intercomparison Projects: Efforts like the Coupled Model Intercomparison Project (CMIP) compare different models to understand their strengths and weaknesses.
- Ensemble Forecasting: Running multiple simulations with slight variations in initial conditions or parameters provides a range of possible future climates, giving an idea of the uncertainty involved.
In conclusion, the accuracy of climate model predictions depends on a multitude of factors ranging from the quality of input data to the inherent complexity of the climate system itself. By continually refining models and incorporating new data, scientists strive to reduce uncertainties and improve the reliability of future climate projections.