Climate models are valuable tools for predicting future climate patterns but come with limitations like uncertainty in initial conditions, emission scenarios, and natural variability. Complexities in model processes, spatial and temporal resolutions, computational constraints, feedback mechanisms, and the representation of human interactions add layers of uncertainty. Validation against historical data is imperfect, and ensemble methods help but do not eliminate all uncertainties. These limitations must be considered when interpreting model predictions to ensure informed decision-making regarding climate change strategies.
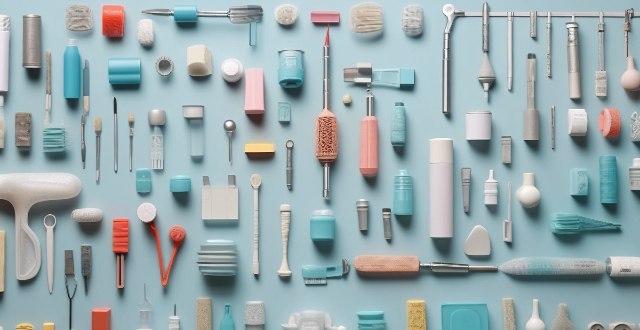
Limitations of Climate Model Predictions
Climate models are powerful tools used by scientists to understand and predict future climate patterns. However, like any scientific model, they have limitations that must be considered when interpreting their predictions. Here are some of the key limitations:
Uncertainty in Model Inputs
- Initial Conditions: Small variations in initial conditions can lead to significantly different outcomes over time, a phenomenon known as "chaotic behavior."
- Emission Scenarios: Predictions rely on estimated greenhouse gas emissions, which are subject to change based on policy decisions, economic growth, and technological advancements.
- Natural Variability: Natural events like volcanic eruptions and solar radiation changes can influence climate but are difficult to predict accurately.
Model Complexity and Resolution
- Simplification of Processes: Models must simplify complex processes, which can lead to discrepancies between modeled and real-world behaviors.
- Spatial Resolution: Coarse resolution may not capture localized effects or small-scale phenomena like coastal upwelling or urban heat islands.
- Temporal Resolution: Short-term fluctuations and extreme events are often not well represented due to the model's time step constraints.
Computational Constraints
- Processing Power: Running multiple high-resolution simulations is computationally expensive and time-consuming.
- Data Storage: Storing vast amounts of output data requires significant resources and can limit the frequency and detail of saved information.
Feedback Mechanisms and Thresholds
- Positive and Negative Feedbacks: The magnitude and timing of feedbacks (like ice-albedo or carbon cycle feedbacks) are uncertain and can amplify or dampen predicted changes.
- Tipping Points: There may be critical thresholds in the climate system that, once passed, could lead to rapid and irreversible changes not easily captured by models.
Representation of Human Interactions
- Socio-economic Factors: Changes in human behavior, such as adaptation measures or shifts in energy use, are challenging to incorporate into models.
- Policy Interventions: International agreements and policy changes can drastically alter emission scenarios but are difficult to predict.
Validation and Historical Data
- Historical Comparisons: While models are tested against past climate data, perfect alignment is rare, and discrepancies raise questions about future projections.
- Ensemble Methods: Using multiple models helps address uncertainty but does not eliminate it entirely.
In conclusion, while climate models provide valuable insights into potential future climates, it is essential to recognize their limitations. These should be communicated alongside predictions to ensure informed decision-making regarding climate change mitigation and adaptation strategies.