Analyzing climate data is a complex task that requires a deep understanding of the subject matter and the use of advanced statistical techniques. Some of the most effective methods for analyzing climate data include time series analysis, regression analysis, spatial analysis, principal component analysis (PCA), and machine learning algorithms. These methods help researchers to identify patterns, trends, and cycles in climate data, determine relationships between variables, analyze data with a geographical component, reduce the dimensionality of large datasets, and identify patterns and trends not apparent through traditional statistical techniques. By using these methods, researchers can gain insights into our changing planet and predict future climate conditions based on current trends.
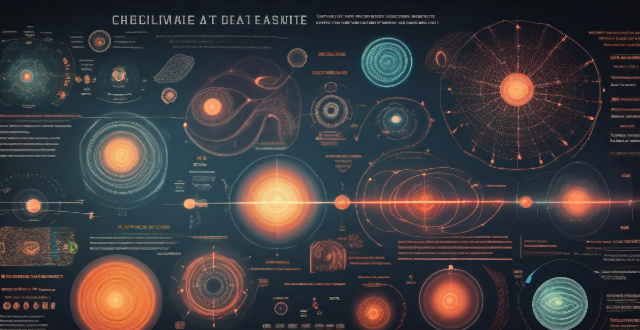
Analyzing Climate Data: Effective Methods
Analyzing climate data is a complex task that requires a deep understanding of the subject matter and the use of advanced statistical techniques. Here are some of the most effective methods for analyzing climate data:
1. Time Series Analysis
Time series analysis is a statistical technique used to analyze data points collected over time. It helps to identify patterns, trends, and cycles in the data. This method is particularly useful for analyzing climate data because it allows researchers to track changes in temperature, precipitation, and other variables over time.
Key Features:
- Identifies patterns, trends, and cycles
- Tracks changes over time
- Helps to predict future climate conditions
2. Regression Analysis
Regression analysis is a statistical technique used to determine the relationship between two or more variables. In climate research, regression analysis can be used to identify how changes in one variable (e.g., carbon dioxide levels) affect another variable (e.g., global temperatures).
Key Features:
- Determines relationships between variables
- Identifies cause-and-effect relationships
- Helps to predict future climate conditions based on current trends
3. Spatial Analysis
Spatial analysis is a method used to analyze data that has a geographical component. This technique is particularly useful for analyzing climate data because it allows researchers to identify patterns and trends across different regions.
Key Features:
- Analyzes data with a geographical component
- Identifies patterns and trends across different regions
- Helps to understand regional variations in climate conditions
4. Principal Component Analysis (PCA)
Principal Component Analysis (PCA) is a statistical technique used to reduce the dimensionality of large datasets while preserving as much information as possible. This method is particularly useful for analyzing climate data because it allows researchers to identify the most important variables driving climate change.
Key Features:
- Reduces dimensionality of large datasets
- Preserves as much information as possible
- Identifies the most important variables driving climate change
5. Machine Learning Algorithms
Machine learning algorithms are powerful tools for analyzing complex datasets. These algorithms can be used to identify patterns and trends in climate data that may not be apparent through traditional statistical techniques.
Key Features:
- Powerful tools for analyzing complex datasets
- Identifies patterns and trends not apparent through traditional statistical techniques
- Helps to predict future climate conditions based on current trends
In conclusion, analyzing climate data requires a combination of advanced statistical techniques and domain knowledge. The methods discussed above are some of the most effective ways to analyze climate data and gain insights into our changing planet.