Machine learning is transforming sports analytics by analyzing player performance, team strategies, and game outcomes. It helps evaluate players' strengths and weaknesses, predict future performance, and develop training plans. Machine learning also aids in understanding how different team strategies affect game outcomes and predicting game results based on various factors. As technology advances, we can expect more innovative applications of machine learning in sports.
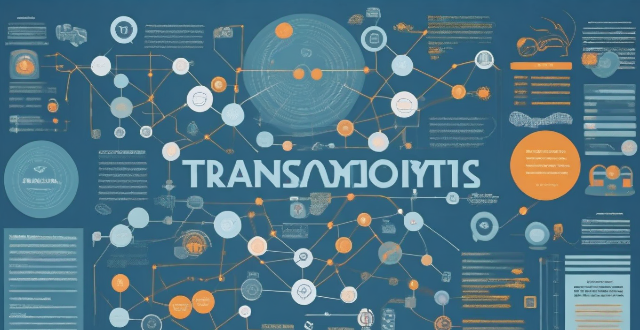
Machine Learning in Sports Analytics
Machine learning plays a significant role in sports analytics by providing valuable insights into player performance, team strategies, and game outcomes. Here are some ways machine learning is used in sports analytics:
Player Performance Analysis
Machine learning algorithms can analyze large amounts of data to identify patterns and trends in player performance. This information can be used to evaluate players' strengths and weaknesses, predict future performance, and develop training plans to improve their skills.
Key Metrics for Player Performance Analysis
- Scoring Statistics: Goals, assists, shots on goal, etc.
- Defensive Statistics: Blocks, interceptions, clearances, etc.
- Physical Attributes: Speed, agility, endurance, etc.
- Technical Skills: Ball control, passing accuracy, shooting accuracy, etc.
Team Strategy Analysis
Machine learning can help coaches and analysts understand how different team strategies affect game outcomes. By analyzing historical data, they can identify which strategies are most effective against certain opponents or in specific situations.
Key Factors for Team Strategy Analysis
- Formation Choices: Offensive vs defensive formations, width vs compactness, etc.
- Tactical Approaches: Direct play vs possession-based football, high pressing vs low block defense, etc.
- Player Roles: Attacking fullbacks vs traditional fullbacks, false nines vs classic strikers, etc.
Game Outcome Prediction
Machine learning models can predict the outcome of a game based on various factors such as team performance, player statistics, weather conditions, and more. These predictions can help teams make informed decisions about lineup selection, substitution timing, and tactical adjustments during the game.
Key Factors for Game Outcome Prediction
- Historical Data: Past match results between teams, recent form, etc.
- Current Form: Recent performances of both teams, injuries/suspensions, etc.
- External Factors: Weather conditions, crowd support, referee biases, etc.
In conclusion, machine learning plays a crucial role in sports analytics by providing valuable insights into player performance, team strategies, and game outcomes. As technology continues to advance, we can expect even more innovative applications of machine learning in the world of sports.