AI technology has revolutionized the way businesses analyze product reviews by providing advanced tools and techniques that enable efficient and accurate analysis. From natural language processing and machine learning to deep learning, AI offers various approaches to extracting valuable insights from customer feedback. By leveraging these technologies, businesses can gain a deeper understanding of customer needs and preferences, identify areas of improvement, and make data-driven decisions that drive growth and success.
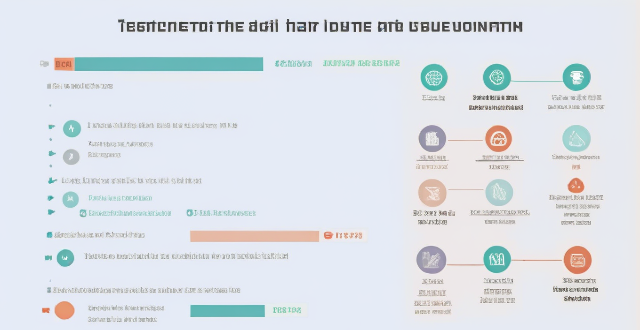
How AI Technology Contributes to Analyzing Product Reviews
Analyzing product reviews is a crucial task for businesses as it helps them understand customer sentiment, identify areas of improvement, and make data-driven decisions. AI technology has revolutionized the way companies approach this task by providing advanced tools and techniques that enable efficient and accurate analysis. In this article, we will explore how AI technology contributes to analyzing product reviews.
Natural Language Processing (NLP)
Natural Language Processing (NLP) is a subfield of AI that focuses on enabling computers to understand, interpret, and generate human language. NLP plays a significant role in analyzing product reviews by helping to extract valuable insights from customer feedback. Here are some ways NLP contributes to analyzing product reviews:
Sentiment Analysis
Sentiment analysis is the process of determining the emotional tone behind a piece of text. NLP algorithms can analyze product reviews and classify them as positive, negative, or neutral based on the words used by customers. This helps businesses gauge overall customer satisfaction and identify areas where they need to improve.
Topic Modeling
Topic modeling is the process of discovering hidden topics within a large corpus of documents. NLP algorithms can analyze product reviews and group them into different topics based on the key phrases and keywords used by customers. This enables businesses to identify common issues or concerns raised by customers and address them accordingly.
Named Entity Recognition (NER)
Named Entity Recognition (NER) is the process of identifying and categorizing named entities in text, such as people's names, locations, organizations, etc. NLP algorithms can analyze product reviews and extract relevant information about the product, such as its features, specifications, and performance. This helps businesses gain a deeper understanding of customer needs and preferences.
Machine Learning (ML)
Machine Learning (ML) is another subfield of AI that involves training algorithms to learn patterns and make predictions based on data. ML contributes to analyzing product reviews by enabling businesses to automate the process and scale their efforts. Here are some ways ML contributes to analyzing product reviews:
Text Classification
Text classification is the process of assigning predefined categories or labels to text documents. ML algorithms can analyze product reviews and automatically categorize them based on their content, such as product quality, delivery time, customer service, etc. This helps businesses quickly identify trends and patterns in customer feedback.
Clustering
Clustering is the process of grouping similar items together based on their characteristics. ML algorithms can analyze product reviews and cluster them into groups based on their similarity, such as products with similar issues or features. This enables businesses to identify common themes and address them collectively.
Anomaly Detection
Anomaly detection is the process of identifying unusual patterns or outliers in data. ML algorithms can analyze product reviews and flag any unusual or suspicious activity, such as fake reviews or spam comments. This helps businesses maintain the integrity of their review system and ensure that customer feedback is genuine.
Deep Learning (DL)
Deep Learning (DL) is a subset of ML that involves training neural networks to perform complex tasks, such as image recognition, speech recognition, and natural language processing. DL contributes to analyzing product reviews by enabling businesses to build more advanced models that can handle large volumes of data and provide more accurate results. Here are some ways DL contributes to analyzing product reviews:
Sentiment Analysis with Neural Networks
Neural networks are a type of machine learning algorithm that mimics the structure and function of the human brain. DL algorithms can analyze product reviews and perform sentiment analysis using neural networks, which can capture more nuanced emotions and context than traditional NLP techniques. This helps businesses gain a more comprehensive understanding of customer sentiment and tailor their products accordingly.
Image Recognition for Visual Reviews
Image recognition is the process of identifying objects, scenes, and activities in images. DL algorithms can analyze product reviews that include images and extract relevant information about the product's appearance, quality, and functionality. This helps businesses monitor visual aspects of their products and address any issues related to packaging, design, or display.
Chatbots for Real-Time Review Analysis
Chatbots are automated programs that use natural language processing and machine learning to interact with users in real-time. DL algorithms can power chatbots that analyze product reviews as they come in and provide businesses with real-time insights and recommendations. This helps businesses respond quickly to customer feedback and make immediate improvements to their products and services.
Conclusion
AI technology has revolutionized the way businesses analyze product reviews by providing advanced tools and techniques that enable efficient and accurate analysis. From natural language processing and machine learning to deep learning, AI offers various approaches to extracting valuable insights from customer feedback. By leveraging these technologies, businesses can gain a deeper understanding of customer needs and preferences, identify areas of improvement, and make data-driven decisions that drive growth and success.