Sports data analysis is the process of collecting, processing, and interpreting sports performance data. The goal is to improve athletic performance through evidence-based decision-making. Key components include data collection, processing, statistical analysis, visualization, and interpretation. Sports data analysis is used in modern sports for performance evaluation, game strategy development, training and preparation, injury prevention and rehabilitation, talent identification and development, fan engagement and media, and business decisions.
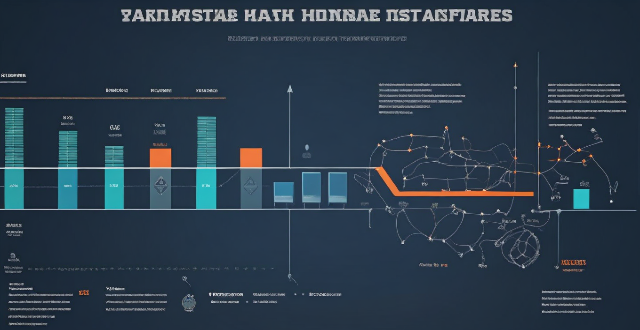
What is Sports Data Analysis?
Sports data analysis, also known as sports analytics or performance analysis, involves the collection, processing, and interpretation of data related to sports performance. This field uses statistical methods to provide insights into team and individual player performance, game strategies, training effectiveness, and injury prevention. The goal of sports data analysis is to improve athletic performance and competitive outcomes through evidence-based decision-making.
Key Components of Sports Data Analysis:
- Data Collection: Gathering raw data from various sources such as wearable technology, video recordings, sensors, and manual input.
- Data Processing: Cleaning and transforming the collected data into a usable format for analysis.
- Statistical Analysis: Applying mathematical models and algorithms to identify patterns, trends, and correlations within the data.
- Visualization: Presenting the analyzed data in visual formats like charts, graphs, and heat maps to facilitate understanding.
- Interpretation: Making sense of the data and its implications for coaching strategies, player development, and overall team management.
How Sports Data Analysis is Used in Modern Sports:
1. Performance Evaluation:
- Player Metrics: Tracking individual metrics like speed, endurance, agility, and technical skills to evaluate player performance.
- Team Statistics: Analyzing team metrics such as possession percentage, shooting accuracy, and passes completed to assess team dynamics.
2. Game Strategy Development:
- Opponent Analysis: Studying opposing teams' tendencies, strengths, and weaknesses to develop effective counter-strategies.
- Play Optimization: Using data to refine playbooks and create tailored game plans that maximize team strengths and exploit opponent vulnerabilities.
3. Training and Preparation:
- Load Management: Monitoring training load to prevent overtraining and reduce the risk of injuries.
- Skill Acquisition: Measuring progress in skill development and adjusting training regimens accordingly.
4. Injury Prevention and Rehabilitation:
- Biomechanical Analysis: Evaluating movement patterns to identify potential injury risks and correct them before they lead to harm.
- Recovery Monitoring: Tracking recovery metrics to ensure athletes are fully rehabilitated before returning to competition.
5. Talent Identification and Development:
- Player Recruitment: Using data to identify promising talent and make informed decisions during the recruitment process.
- Youth Development: Analyzing young athletes' performance data to tailor development programs that maximize their potential.
6. Fan Engagement and Media:
- Enhanced Broadcasting: Providing viewers with real-time statistics and analytical insights during broadcasts.
- Interactive Experiences: Offering fans engaging content through apps and social media that utilize player and game data.
7. Business Decisions:
- Sponsorship Value: Determining the value of sponsorship deals based on player performance data and marketability.
- Fan Analytics: Analyzing fan behavior and preferences to inform marketing strategies and improve the overall fan experience.
In summary, sports data analysis has become an integral part of modern sports, offering valuable insights that can significantly impact performance, strategy, training, health management, talent identification, fan engagement, and business decisions across all levels of athletic competition.