The text discusses the limitations of current climate prediction methods, including model uncertainty, natural variability, scenario dependence, data availability and quality, and computational resources. These limitations can impact the accuracy and reliability of predictions, making it difficult to develop effective strategies for mitigating the effects of climate change. Addressing these challenges will be essential for developing more accurate and reliable climate predictions that can support effective decision-making and adaptation strategies in response to climate change.
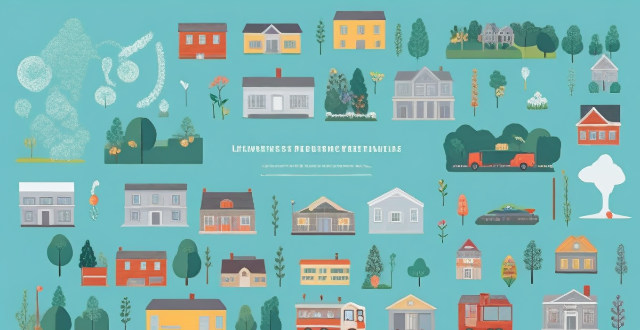
Limitations of Current Climate Prediction Methods
Climate prediction is a complex and challenging field that involves the use of various models and techniques to forecast future climate conditions. While significant progress has been made in recent years, there are still several limitations associated with current climate prediction methods. These limitations can impact the accuracy and reliability of predictions, making it difficult to develop effective strategies for mitigating the effects of climate change.
Model Uncertainty
One of the primary limitations of climate prediction methods is model uncertainty. Climate models are mathematical representations of the Earth's climate system, and they rely on a wide range of input data and assumptions about how the system works. However, due to the complexity of the climate system, there is always some degree of uncertainty associated with these models. This uncertainty can arise from factors such as:
- Incomplete or imperfect understanding of physical processes
- Limited availability of high-quality observational data
- Variability in model parameters and initial conditions
As a result, different climate models may produce different predictions for the same scenario, which can make it challenging to determine the most accurate or reliable forecast.
Natural Variability
Another limitation of climate prediction methods is natural variability. The Earth's climate system is inherently variable, with many factors contributing to fluctuations in temperature, precipitation, and other variables over time. Some examples of natural variability include:
- El Niño Southern Oscillation (ENSO) events
- Solar radiation changes
- Volcanic eruptions
- Ocean currents and circulation patterns
These natural variations can have a significant impact on climate predictions, particularly when attempting to forecast short-term changes or localized events. While climate models attempt to account for these factors, their influence can be difficult to predict accurately, leading to uncertainties in climate projections.
Scenario Dependence
Climate predictions are often based on scenarios that describe potential future greenhouse gas emissions, land use changes, and other human activities that can influence climate change. These scenarios are developed using a combination of expert judgment and statistical modeling techniques, but they are inherently subjective and dependent on assumptions about future socioeconomic developments and policy decisions. As a result, climate predictions can vary significantly depending on the scenario chosen, which can make it difficult to compare results across different studies or to develop targeted adaptation strategies.
Data Availability and Quality
Climate prediction methods rely heavily on observational data to inform model development and validation. However, there are often gaps in the available data, particularly for regions where monitoring networks are limited or nonexistent. Additionally, the quality of existing data can be influenced by factors such as measurement errors, instrument calibration issues, and changes in observational practices over time. These challenges can limit the accuracy and precision of climate predictions, particularly for variables that are difficult to measure or for regions where data coverage is poor.
Computational Resources
Finally, climate prediction methods require significant computational resources to run complex models and perform large-scale simulations. While advancements in computing technology have enabled more detailed and sophisticated modeling efforts, these calculations remain resource-intensive and time-consuming. As a result, climate predictions can be limited by the availability of computational power and funding for research projects.
In conclusion, while current climate prediction methods have made significant strides in improving our understanding of future climate conditions, they still face numerous limitations related to model uncertainty, natural variability, scenario dependence, data availability and quality, and computational resources. Addressing these challenges will be essential for developing more accurate and reliable climate predictions that can support effective decision-making and adaptation strategies in response to climate change.